Turning AI Insights into Action with MaestroQA
AI-powered insights have the potential to revolutionize how businesses handle customer interactions, but insights alone are not enough—action is what drives real change. Many organizations struggle with operationalizing AI data, leaving valuable insights trapped in dashboards and reports.
This guide shows how MaestroQA helps businesses bridge the gap between insight and action by creating scalable QA processes that integrate AI-driven automation with human-led calibration. With automation, context-driven analysis, and continuous improvement, businesses can transform AI-powered outputs into meaningful business results.
Challenges of Operationalizing AI Insights
Despite AI’s potential, organizations often struggle to turn AI-driven insights into operational outcomes. Successfully integrating AI into business processes requires addressing several common obstacles:
Common Obstacles
- Even with AI flagging tickets, high volumes of data can overwhelm teams without clear workflows.
- Teams may lack structured processes to filter, prioritize, and address flagged issues effectively.
- AI models may misclassify issues if prompts are too narrow or definitions are unclear.
- Misclassifications waste time and require manual audits to adjust AI models and refine detection criteria.
- Data stuck in dashboards or reports fails to drive change unless integrated into active QA processes.
- Insights must be surfaced through actionable dashboards, automated alerts, and clear next steps.
- Without consistent interpretation of AI outputs, teams can struggle to define what qualifies as a complaint or priority issue.
- Ambiguous definitions of key terms like “complaint” can create discrepancies in classification.
- AI-driven processes need continuous human input to refine prompts, retrain models, and close performance gaps.
- Without structured feedback loops, recurring issues remain unresolved, limiting long-term improvement.
By addressing these challenges with MaestroQA’s platform, businesses can operationalize AI-powered outputs through well-defined workflows that turn insights into real-world improvements.
Building a Human and AI-Powered QA Framework
While AI can process massive datasets and identify patterns faster than humans, it lacks the nuanced understanding needed for complex customer interactions. Human QA teams bring context, empathy, and domain-specific expertise to interpret AI-driven findings accurately.
With MaestroQA’s platform, businesses can combine AI’s automation and scalability with human insight by:
Reducing Manual Effort: AI automates repetitive tasks, while MaestroQA routes flagged tickets to human reviewers for deeper analysis.
Increasing Accuracy: Teams refine AI models using MaestroQA’s RCA rubrics and targeted audits.
Driving Better Outcomes: Human-driven validation ensures AI insights are relevant and actionable.
Example: How AI and QA Teams Collaborate for Better Outcomes
To fully operationalize AI-powered QA, AI and QA teams must collaborate through clear, structured steps.
Below is an example of how this process works within MaestroQA’s AI Platform
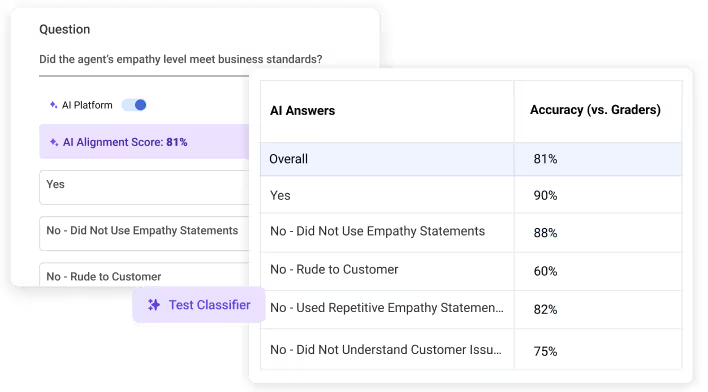
- Custom filters identify specific tickets based on defined criteria.
- AI scans the selected tickets based on a custom prompt.
- AI generates outputs for each ticket based on the provided prompt and business specific context
- Humans grade the AI-generated results and receive an alignment score, showing how closely AI decisions match human expectations.
- Teams adjust the context provided to the LLM (e.g., refining the prompt or adding clarifications to business-specific context) and rerun tests to improve accuracy.
Once a high level of accuracy is achieved with the LLM, AutoQA scales this process across 100% of tickets.
Steps for Implementing an AI-Powered QA Workflow
- Configure classifiers with precise definitions for complaints or compliance risks.
- Set tracking metrics like complaint validation rates and agent escalation trends.
- Manual QA Audits Teams validate flagged tickets and document findings.
- Iterative Model Training Tech teams adjust AI prompts based on validated feedback.
- Automated Reporting Dashboards track both AI-driven insights and manual validations.
- Coaching and Action Plans Managers receive tailored coaching recommendations.
Best Practices for Success
Start Small, Scale Fast: Begin with one workflow before full deployment.
Collaborate Cross-Functionally: Align QA and tech teams early.
Iterate Continuously: Use RCA findings to refine AI models and processes regularly.
Real World Example: A Fintech Company’s AI-Powered QA Process

Implementing AI-powered QA can transform how organizations handle large-scale customer interactions. Consider how a fintech company operationalized AI-driven insights using MaestroQA. By blending automated ticket classification with human QA reviews, they resolved key operational challenges, improved complaint management, and built a continuous improvement system driven by actionable data.
Challenges Faced
The company faced challenges in scaling its customer complaint handling process due to high ticket volumes and inconsistent classifications. To address this, they implemented an AI-powered QA framework that combined automated classification with human-led reviews.
Key Phases of Implementation
AI Metric Setup
The company configured an AI Metric to detect potential complaints by scanning customer tickets for specific keywords and patterns.
Human-Led Audits
A dedicated QA team calibrated with the AI outputs in the AI Platform, verifying whether the tickets truly reflected customer dissatisfaction based on internally defined criteria.
Feedback Loop for Model Refinement
- QA team findings were shared with tech teams to refine AI platform prompts.
- Frequent workshops were conducted to adjust definitions and improve AI output accuracy.
Operational Workflows and Dashboards
- Customized dashboards tracked complaint validation rates and root causes.
- Team leads reviewed flagged cases to understand missed escalations and agent behavior trends.
Results Achieved Through MaestroQA
Complaint identification accuracy rose by 18% after multiple refinement cycles.
Insights gathered helped team leads coach agents more effectively, reducing unresolved complaints.
The team continuously iterated on AI model prompts, reducing false positives and enabling faster complaint resolutions.
Ready to Elevate Your AI Strategy?
Combining AI-powered insights with human QA expertise unlocks actionable data, drives team accountability, and fosters continuous operational improvement. Use this guide to build scalable QA workflows that transform raw AI data into meaningful business outcomes.
Take the next step by exploring how our platform can drive performance, enhance customer satisfaction, and streamline your QA processes, contact us today!