- Deploy AI Queries: Use custom, GPT-powered AI queries to explore trends and validate or refine your hypothesis.
- Human RCA: Assign targeted RCA tasks to Graders, enabling them to analyze cases and identify key trends and actionable insights.
Building a Path from QA to Performance Excellence
Performance excellence goes beyond hitting targets—it's about refining the processes that drive results. MaestroQA equips you with the insights and tools to identify improvement areas and make data-driven decisions that elevate team performance.
The Vision
Moving from Random to Targeted QA
Random QA, while historically useful, often lacks the depth needed to drive meaningful improvements. It’s reactive, addressing issues after they’ve occurred rather than preventing them in the first place. Our vision is to shift to targeted QA, where evaluations are driven by data, helping teams prevent issues before they arise. This proactive approach leads to higher customer satisfaction and greater operational efficiency.
From Vision to Practice
Two paths to Targeted QA
We’ve outlined two paths to implement targeted QA within your organization. These paths provide a roadmap for using data and AI to transform your QA processes.
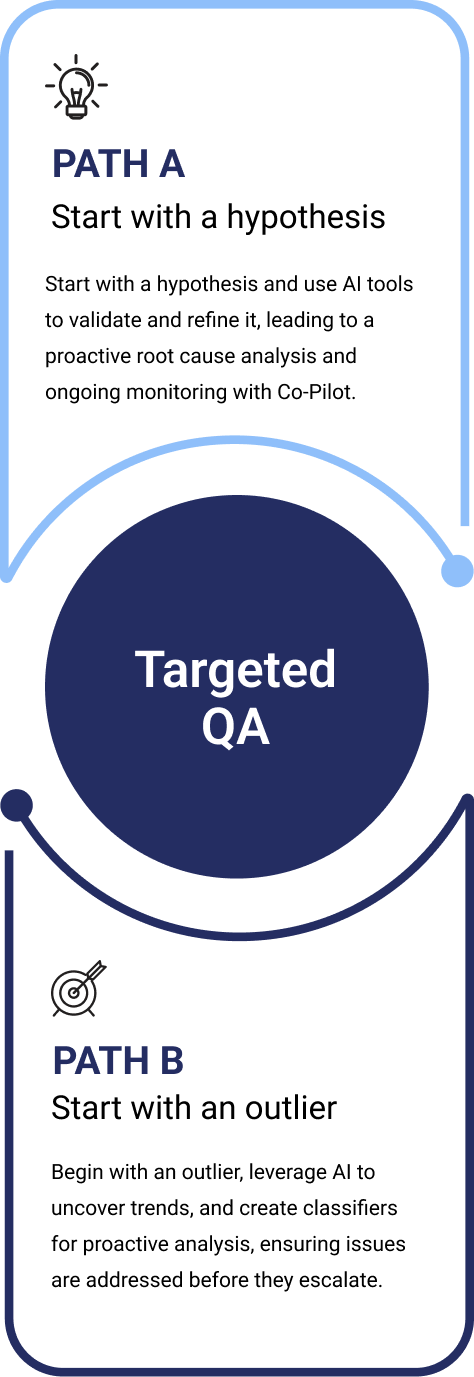

Hypothesize: Start with an idea or anecdotal evidence that something might be amiss—perhaps you’ve noticed a pattern in customer complaints or an uptick in escalations.
Leverage Conversation Analytics: Utilize Conversation Analytics to track behavioral trends, allowing you to generate reports that drill down from overall metrics to individual conversations.
Root Cause Analysis (RCA): Conduct RCA using MaestroQA’s RCA workflows. Dig into specific cases identified by your AI metrics and complete a Root Cause sprint that can surface insights you might not have considered.
Path A in Action
Challenge
Customer thinks a recent increase in escalations is being caused by customer confusion about new billing policies.
Objective
Determine if escalation increases are being caused by the updated billing policies.
Approach
- Hypothesis: Potential confusion over new billing terms.
- Leverage Conversation Analytics: Used MaestroQA’s Custom Metric Builder to build a KPI that identifies the root causes of billing escalations.
- RCA: Conducted RCA using both of MaestroQA’s RCA workflows.
- AI Queries: Queried KPI data using custom GPT-powered AI prompts to uncover themes and trends related to billing escalations, pinpointing communication gaps.
- Human RCA: Conducted a targeted RCA sprint, where graders analyzed escalated tickets and identified agent training needs.
Solution
- Agent Training: Implemented targeted training sessions focusing on the new billing policies.
- Customer Education: Updated and distributed customer-facing materials.
- Policy Updates: Clarified ambiguous terms in the billing policy to reduce confusion.
Results
- Resolution Rate: 35% decrease in billing-related escalations within one month.
- CSAT: 12% increase in customer satisfaction for billing inquiries.
- Agent Performance: 20% reduction in average handling time (AHT).

Identify the Outlier: Begin by spotting an outlier on your performance dashboard—something that stands out and needs further investigation.
Root Cause Analysis (RCA): Conduct RCA using MaestroQA’s RCA workflow. Dig into specific cases identified by your AI metrics and complete a Root Cause sprint that can surface insights you might not have considered.
- Query with AI: Use custom, GPT-powered AI queries to explore trends and validate or refine your hypothesis.
- Human RCA: Assign targeted RCA tasks to Graders, enabling them to analyze cases and identify key trends and actionable insights.
Build a Custom Metric: If your RCA reveals an issue you want to track, create a custom KPI in Maestro to track it across all interactions, helping you determine if the problem is isolated or systemic.
Path B in Action
Challenge
A sudden 10% drop in CSAT scores with no obvious cause, leading to concerns about a broader customer satisfaction issue.
Objective
Identify the root cause of the sudden dip in CSAT scores and address it to restore customer satisfaction.
Approach
- Outlier Identification: Detected an unexpected drop in CSAT scores on the performance dashboard, without any immediate or clear reasons.
- RCA: Conducted RCA using MaestroQA’s RCA workflows to uncover the cause of the CSAT dip.
- Query with AI: Used GPT-powered AI queries to analyze customer feedback and support interactions, identifying confusion around recent product UI changes as a contributor.
- Human RCA: Conducted a focused RCA sprint where graders analyzed flagged cases, uncovering that misaligned user expectations with the new UI were a primary cause of DSAT tickets.
- Build a Custom Metric: Created a custom KPI in Maestro to track UI-related issues across all interactions, allowing the team to monitor and determine that this was an isolated issue.
Solution
- Agent Training: Targeted training for agents on addressing new UI concerns.
- Customer Education: Updated documentation and in-app tutorials on UI changes.
- Feature Adjustment: Tweaked the UI for better intuitiveness and user experience.
Results
- Ticket Volume: 20% reduction in tickets related to the UI within two weeks.
- CSAT Recovery: CSAT scores rebounded by 8% within one month after implementing these changes.
- User Engagement: 12% increase in user engagement with the updated product features.
Why Targeted QA is the Future
Random QA isn’t as impactful as a targeted approach. Targeted QA allows you to focus on what matters most, leveraging data and AI to make smarter, more informed decisions. With this approach, you’re not just reacting to problems—you’re anticipating them and taking steps to prevent them.
MaestroQA’s platform is designed to support this transition, providing you with the tools and insights needed to make QA a strategic asset. By embracing targeted QA, you can transform your business operations, ensuring that every workflow is optimized.
MaestroQA Custom Metric Builder
Tailored Metrics for Comprehensive Analysis
Build custom metrics to suit your team’s workflows and use cases, analyzing 100% of tickets to uncover root causes and trends—all integrated into your Performance Dashboard.
Phrase Match Metrics: Define words and phrases to be identified across 100% of tickets
LLM Metrics: Define business-specific criteria to be AI-analyzed across all interactions
Process Based Metrics: Define business processes to be analyzed across 100% of tickets
FAQs for Success with Targeted QA
What’s the difference between random and targeted QA?
Random QA often checks general performance without focusing on specific insights, while targeted QA uses data to focus on high-impact areas, allowing for more analytical, in-depth evaluations.
How can AI impact headcount in a QA team?
A common misconception is that AI will directly reduce QA headcount. Instead, AI supports auditing by automating processes, which helps the team focus more on targeted, high-impact QA. While AI can streamline some tasks, it doesn't replace the need for skilled team members who can provide critical analysis and data-driven insights.
What skills are most valuable for a targeted QA team?
Transitioning to targeted QA requires team members with critical thinking and analytical skills. It’s recommended to look for people with customer empathy and problem-solving experience rather than strictly QA backgrounds.
What should be considered when evolving a QA team structure for targeted QA?
Focus on creating a structure that enables targeted QA to maximize insights and results. Seek team members with critical thinking, curiosity, and customer empathy—qualities often found in top-performing agents who understand your processes and bring a fresh analytical perspective.
How concerns about moving from random to targeted QA in BPO contracts be addressed?
Some BPO contracts rely on QA scores, which can complicate a shift to targeted QA. Consider meeting minimum contract requirements for random auditing while focusing additional resources on targeted QA for more actionable insights.
How often should AI-driven insights and metrics be validated?
Regular validation, ideally every 30 to 45 days, is recommended to ensure accuracy. As AI models can drift, periodic spot checks and adjustments are necessary to maintain reliable insights.
How long does it take to fully transition to targeted QA and build out new capabilities in MaestroQA?
Transition time can vary based on company size and complexity. For foundational setup, three to six months is typical, but targeted insights can be achieved more quickly through focused sprints on high-priority areas.
Should there be separate teams for traditional QA tasks and new targeted QA initiatives?
It’s beneficial to have one team focused on traditional QA tasks and another on developing targeted QA practices and using MaestroQA’s advanced tools. This helps balance immediate needs with longer-term goals.
Ready to Elevate Your AI Strategy?
The full guide includes in-depth FAQs, case studies, and practical steps to help your team excel in targeted QA. Get the guide now!
Guide
Unlock Your Path From QA to Performance Excellence
From Random QA to Targeted Insights
Discover how targeted, data-driven strategies can transform your team's performance. Download our exclusive guide to discover the tools and actionable steps that will redefine how you approach QA.
